Refinement of DeepLabV3+ for Enhanced Crack Detection in Port Infrastructure
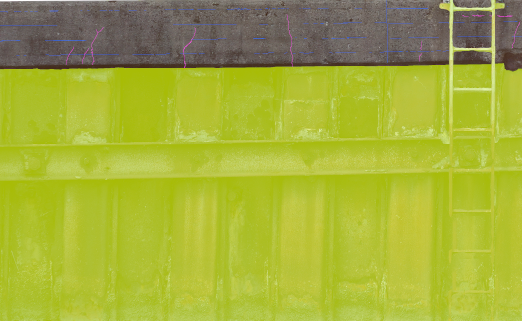
Betreuung: | Marvin Scherff, Hamza Alkhatib |
Bearbeitung: | Mohd Adeeb Khan |
Jahr: | 2023 |
Datum: | 19-01-24 |
Laufzeit: | 11/2023 - 04/2024 |
Ist abgeschlossen: | ja |
The ever-growing demand for maintaining the structural integrity of port infrastructure calls for advancements in automated inspection processes. With the advent of the Port AI project, the horizon of sensor platforms and intelligent software to scrutinize port infrastructures has significantly broadened. The integration of these advancements into Building Information Modeling (BIM) systems is poised to revolutionize the field of structural health monitoring. This master thesis aims to contribute to this transformative journey by focusing on image-based crack detection, a critical aspect of infrastructure inspection.
The primary goal of this thesis is to customize the well-established DeepLabV3+ segmentation network to accurately detect the specific attributes of crack formations. The iteration currently in use is fine-tuned predominantly for identifying corrosion, trained on a proprietary dataset featuring corrosion damage from Wilhelmshaven’s container port. The subtle and frequently indistinguishable color patterns of cracks introduce a set of challenges not encountered with the typically more evident corrosion damage. Addressing these challenges will involve an intensive review and modification of the network architecture and the training processes. The task includes pinpointing the optimally configured model and rigorously comparing its performance with that of a previously established UNet-based segmentation network within the Port AI project. Furthermore, the research will develop ensemble-based uncertainty maps, empowering the engineering personnel to make informed corrections to the predictive outputs. A cooperating engineering office has provided a robust dataset for this project, which includes meticulously annotated ground truth images representing the categories of paramount importance to routine inspection activities.